Artificial General Intelligence (AGI) represents a future where machines achieve human-level autonomy and intelligence, revolutionizing industries from healthcare to manufacturing. Although AGI is still a theoretical concept, its potential to transform society is immense. As we approach this possibility, organizations must start preparing by building robust data infrastructures and fostering seamless human-AI collaboration.
Key Takeaways:
• AGI could revolutionize industries by enabling machines to perform tasks with human-like intelligence, adaptability, and creativity, far surpassing current AI capabilities.
• Organizations should begin preparing for AGI by leveraging existing AI technologies, building strong data-driven infrastructures, and adopting collaborative AI platforms.
• Achieving AGI requires overcoming significant challenges in areas like visual and audio perception, fine motor skills, problem-solving, and social engagement. Various approaches, including symbolic AI, neural networks, and whole brain emulation, are being explored to realize AGI’s potential.
• While AGI remains theoretical, businesses can benefit from current AI advancements by developing robust AI strategies and integrating AI into their operations, laying the groundwork for future AI developments.
Artificial General Intelligence and Its Potential Impact on Society
Imagine a world where machines aren’t just tools executing pre-programmed tasks but entities operating with the autonomy and competence of human minds. In this world, computer intelligence drives self-piloting cars, pioneers groundbreaking scientific discoveries, delivers tailored customer experiences, and even ventures into uncharted territories.
This is the promise of artificial general intelligence (AGI)—a transformative technology that could revolutionize every facet of human life and work. While AGI is still a theoretical concept, its potential impact is profound. Organizations should begin preparing now by building robust data infrastructures and fostering environments where humans and AI collaborate seamlessly.
AGI, often referred to as “strong AI,” envisions a future where machines achieve human-level learning, perception, and cognitive flexibility. Unlike humans, AGIs would never tire or have biological limitations, continuously learning and processing information at speeds beyond our imagination. The development of such synthetic minds, capable of solving complex problems, could disrupt and redefine entire industries, taking over tasks that were once the exclusive domain of human intelligence.
Picture an AGI-controlled self-driving car—not just navigating unfamiliar roads but engaging in real-time, personalized conversations with its passengers. It could answer questions about local culture, recommend restaurants based on preferences, and even recall details from past trips to enhance the experience further. This level of interaction and adaptability goes far beyond what today’s AI systems can offer.
Current AI technologies, like GPT-4o, Gemini 1.5 Pro and Claude Sonnet 3.5, are already demonstrating remarkable abilities in generating human-quality text, performing specific tasks, translating languages, and creating diverse content. However, while these large language models (LLMs) might seem advanced, they are not the sentient, thinking machines of science fiction.
These AI systems achieve their feats through a blend of sophisticated algorithms, natural language processing (NLP), and computer science principles. Trained on vast amounts of text data, LLMs like ChatGPT recognize patterns and statistical relationships in language. NLP techniques enable them to understand the nuances of grammar, syntax, and context. By leveraging complex algorithms, these systems generate human-like text, accurately translate languages, and produce creative content in various styles.
Today’s AI, including generative AI, excels at processing massive datasets, automating workflows, and generating human-like text—this is “narrow AI.” Despite its impressive capabilities, narrow AI lacks true understanding and cannot adapt to situations beyond its training. This stark limitation underscores the immense gap between current AI and the potential of AGI.
The leap from narrow AI to true AGI represents one of the most significant challenges in AI research. Scientists are delving into areas like artificial consciousness, general problem-solving, and common-sense reasoning within machines. While the timeline for achieving AGI is uncertain, organizations can start preparing for this future by establishing a strong, data-driven infrastructure today. This groundwork will be essential to harnessing the power of AGI when it arrives.

How Can Organizations Prepare for AGI?
Preparing for Artificial General Intelligence (AGI) is challenging due to its theoretical nature, but organizations can take proactive steps by leveraging existing AI technologies and infrastructure. If AGI development follows a path similar to narrow AI, some current tools and technologies will likely play a crucial role in its adoption.
The concept of general intelligence in AGI is still a topic of intense debate among AI researchers. Some experts suggest that AGI would possess self-awareness and self-regulation, with capabilities strikingly close to human-level performance. However, current AI advancements, while impressive, are categorized as narrow AI—highly specialized systems that excel in specific tasks but lack the general problem-solving abilities envisioned for AGI.
Despite the uncertainty surrounding AGI’s timeline, which ranges from 2030 to 2050 or beyond, it’s essential for organizations to manage expectations and focus on maximizing the value of today’s AI applications. While some leaders express reservations about the benefits of current AI, many organizations are already heavily investing in generative AI (gen AI) deployment, significantly increasing budgets, expanding use cases, and transitioning projects from experimentation to full production.
In 2023, companies reported spending an average of $7 million on foundation model APIs, self-hosting, and fine-tuning models. Nearly all respondents noted promising early results from gen AI experiments and planned to boost their spending in 2024 to support production workloads. Interestingly, there’s a shift in funding from innovation budgets to software line items, indicating that generative AI is quickly becoming a core technology.
On a more tactical level, some organizations are reallocating generative AI budgets toward headcount savings, especially in customer service. One company reported saving around $6 per call by using a large language model (LLM) powered customer service system, resulting in a 90% cost reduction—a compelling reason for increased investment in gen AI.
Beyond cost savings, organizations are also focused on measuring the return on investment (ROI) of gen AI, looking at factors like revenue generation, efficiency gains, and accuracy improvements. A notable trend is the adoption of a multi-model approach, where organizations use multiple AI models together to combine strengths, tailor solutions to specific needs, avoid vendor lock-in, and capitalize on rapid advancements in the field.
In 2024, 46% of survey respondents showed a preference for open-source models such as llama 3 and Mistral. While cost wasn’t the primary factor, it reflects a growing belief that the value generated by gen AI justifies the investment. This shift illustrates that executives increasingly prioritize accuracy and effectiveness over cost.
While enterprises remain interested in customizing AI models, the rise of high-quality open-source models has led many to opt for fine-tuning existing models rather than training new LLMs from scratch. Techniques like retrieval-augmented generation are becoming popular for tailoring these models to specific organizational needs.
Most enterprises (72%) that access models via APIs use those hosted on their cloud service providers. Additionally, applications that integrate LLMs with other technologies to create comprehensive solutions and rethink enterprise workflows are performing well in the market.
A study explored the value generated by generative AI across various business functions. Key areas of ROI include:
Text (83%): Gen AI is automating tasks like report writing, document summarization, and marketing copy generation.
Code (62%): Gen AI helps developers write code more efficiently and with fewer errors.
Audio (56%): Gen AI-powered call centers use realistic audio to assist customers and employees.
Image (55%): Gen AI can simulate product appearances in customer settings or reconstruct accident scenes for insurance assessments.
Other Areas: Video generation (36%) and 3D model generation (26%) are used for creating marketing materials, virtual renderings, and product mockups.
One of the significant hurdles in generative AI development is the skills gap. Startups that offer tools to simplify in-house gen AI development are likely to see faster adoption, as acquiring the right talent remains a challenge for many enterprises.
While AGI promises a level of machine autonomy far beyond what gen AI offers, even the most advanced AI systems still require human expertise. Building an in-house team with skills in AI, deep learning, machine learning (ML), and data science is crucial. No matter how advanced AI becomes, data scientists, AI engineers, computer scientists, and ML specialists will remain essential for developing and deploying these systems.
As AI technology continues to evolve, so will its use cases. By focusing on these foundational areas, organizations can position themselves to harness the power of AI advancements as they emerge.
Improving AI to Reach AGI
While artificial intelligence (AI) has made remarkable progress, achieving Artificial General Intelligence (AGI)—machines with human-level intelligence—remains a daunting challenge. Here are seven critical areas where current AI falls short and where AGI would need to excel:
Visual Perception: Despite advances in computer vision, including facial recognition and object detection, AI still lags behind human visual capabilities. It struggles with understanding context, accurately perceiving color, and interpreting partially obscured objects. AGI would need to possess a human-like ability to interpret complex visual scenes, understand context, and adapt to varying visual conditions.
Audio Perception: While AI has made strides in speech recognition, it often fails to understand accents, sarcasm, and emotional tones in speech. It also struggles with filtering out background noise and interpreting non-verbal cues like sighs, laughter, or changes in volume. AGI must reliably understand a wide range of speech patterns, tones, and non-verbal expressions, adapting to subtle nuances in human communication.
Fine Motor Skills: Although AI paired with robotics has seen success in certain tasks, it lacks the fine motor skills needed for delicate and complex manipulations, such as handling fragile objects or quickly adapting to new physical tasks. AGI, in conjunction with robotics, would need to master delicate and precise motor skills, enabling it to manipulate tools and objects in real-world environments with human-like dexterity.
Problem-Solving: Narrow AI excels at solving specific, well-defined problems but lacks the general reasoning and critical thinking required to address novel, complex issues. AGI would need the ability to solve problems like a human, using reasoning, critical thinking, and the ability to make decisions even with incomplete information.
Navigation: Self-driving cars demonstrate impressive capabilities, but AI still struggles with navigating complex, unpredictable environments that humans manage with ease, such as crowded streets or uneven terrain. AGI must navigate diverse and dynamic environments with the same adaptability and awareness that humans exhibit, responding to unexpected challenges in real-time.
Creativity: While AI can generate creative content to some extent, it lacks the originality and novelty that define true creativity. AI’s outputs are often derivative, based on patterns learned from existing data. AGI would need to demonstrate genuine creativity, generating new ideas, concepts, and solutions that are original and innovative, akin to human creative processes.
Social and Emotional Engagement: Human intelligence is deeply rooted in social and emotional interactions, an area where AI still struggles. AI has limited ability to recognize and interpret emotions, whether in facial expressions, body language, or tone of voice. AGI would need to understand and appropriately respond to human emotions, adjusting its communication and behavior based on the emotional state of others.
These skills represent the significant hurdles that AI must overcome to evolve into AGI, marking the frontier of current AI research and development.
AGI Examples
Once theoretical AGI overcomes current challenges and becomes a reality, its applications could transform numerous industries. Here are some examples of how AGI might revolutionize various fields:
Customer Service
An AGI-powered customer service system could revolutionize how businesses interact with their customers. By accessing vast amounts of customer data and integrating real-time analytics, AGI could deliver highly efficient and personalized service. Imagine a system that creates detailed customer profiles, including demographics, past interactions, needs, and purchasing habits. With this information, AGI could anticipate potential issues, tailor responses, suggest solutions, and even predict follow-up questions.
Example: Picture the best customer service experience you’ve ever had—AGI could replicate and surpass this by using advanced perception systems to anticipate problems before they arise. It could analyze the tone of a customer’s voice to gauge their mood, recall intricate details from previous interactions, and engage in meaningful conversations that address complex issues. With its ability to understand the nuances of human language and emotions, AGI would adapt its communication style to be empathetic and supportive, ensuring a positive and effective customer experience.
Coding Intelligence
AGI could also revolutionize software development by understanding the logic and intent behind existing codebases. Beyond merely analyzing code, AGI could suggest improvements, generate new code based on specific human requirements, and enhance productivity by comprehending the architecture, dependencies, and change history of complex systems.
Example: Imagine a programmer working on an e-commerce platform who needs a function to calculate shipping costs based on location, weight, and delivery method. The programmer could simply instruct AGI, “Create a function for calculating shipping costs.” AGI would analyze the relevant code, generate a draft function complete with comments explaining the logic, and allow the programmer to review, optimize, and integrate it seamlessly. This level of coding intelligence could drastically reduce development time and improve code quality.
Navigation, Exploration, and Autonomous Systems
Current self-driving cars and autonomous systems depend heavily on pre-programmed maps and sensors. However, AGI would take autonomy to the next level by not only perceiving its surroundings but truly understanding them. An AGI system could analyze real-time data from cameras, LiDAR, and other sensors to identify objects, assess risks, and anticipate environmental changes, such as sudden weather shifts or unexpected obstacles. Unlike current systems that have limited response capabilities, AGI would be capable of making complex, real-time decisions.
AGI might consider multiple variables simultaneously, such as traffic flow, weather conditions, and potential hazards beyond the immediate sensor range. Unlike today’s systems, which follow pre-programmed routes, AGI-powered systems would learn from experience, adapt to new situations, and even explore uncharted territories. Imagine AGI guiding autonomous exploration vehicles through intricate cave systems or drones assisting in search and rescue missions in dynamic and unpredictable environments.
Example: An AGI-powered self-driving car encounters an unexpected traffic jam on its usual route. Rather than rigidly adhering to pre-set instructions, the AGI analyzes real-time traffic data from other connected vehicles and identifies alternative routes. It considers various factors such as distance, travel time, and potential hazards like construction zones. The AGI then selects the safest and most efficient route, ensuring a smooth and informed experience for the passengers.
Healthcare
The vast amounts of medical data generated today often remain underutilized. AGI could revolutionize healthcare by analyzing medical images, patient records, and genetic data to detect subtle patterns that might be missed by human practitioners. By leveraging historical data and medical trends, AGI could predict a patient’s risk of developing specific diseases and tailor treatment plans to the individual’s genetic makeup and medical history. This personalized approach could result in more effective therapies with fewer side effects.
Example: A patient presents with concerning symptoms, prompting the doctor to upload their medical history and recent test results to an AGI-powered medical analysis system. The AGI quickly analyzes the data and identifies a rare genetic mutation linked to a particular disease. This critical information enables the doctor to make a targeted diagnosis and develop a personalized treatment plan, potentially improving the patient’s outcome significantly.
Education
Imagine an AGI tutor that doesn’t just present information but tailors the entire learning experience to each individual student. AGI could analyze a student’s performance, learning style, and knowledge gaps to craft a customized learning path. It wouldn’t apply a one-size-fits-all approach; instead, AGI would adjust the pace and difficulty of material in real time based on the student’s comprehension. If a student struggles with a concept, the AGI might offer alternative explanations, examples, and interactive simulations. For those who quickly grasp a topic, it could introduce more advanced material to keep them challenged and engaged. Beyond traditional teaching methods, AGI could create immersive simulations, personalized exercises, and gamified experiences that make learning more engaging and motivating.
Example: A student is having difficulty with a complex math concept. The AGI tutor recognizes this and adapts its teaching strategy. Rather than relying on a standard lecture, it visually represents the concept through interactive simulations, breaking it down into simpler, more digestible steps. The student practices with exercises specifically designed to address their unique knowledge gaps, with the AGI providing ongoing feedback and encouragement to keep them motivated.
Manufacturing and Supply Chain Management
AGI could revolutionize manufacturing by optimizing every step of the production process. By analyzing vast amounts of data from sensors across the production line, AGI could identify bottlenecks and suggest real-time adjustments to machine settings, optimizing production schedules for maximum efficiency. Furthermore, AGI could analyze historical data and sensor readings to predict equipment failures before they occur, enabling a proactive approach that prevents costly downtime and ensures smooth operations. In supply chain management, AGI could manage complex logistics networks in real time, optimizing delivery routes, predicting potential delays, and adjusting inventory levels to ensure just-in-time delivery, thereby minimizing waste and reducing storage costs.
Example: Picture an AGI system monitoring a factory assembly line. It detects a subtle vibration in a critical machine, indicating potential wear and tear. By analyzing historical data, the AGI predicts a likely failure within the next 24 hours and alerts maintenance personnel, allowing them to address the issue proactively. This intervention prevents a breakdown and ensures that the production line continues to run smoothly, avoiding costly downtime.
Financial Services
AGI has the potential to revolutionize financial analysis by surpassing traditional methods and uncovering insights that human analysts might miss. By analyzing vast datasets that include financial news, social media sentiment, and even satellite imagery, AGI could identify complex market trends and potential disruptions with unprecedented accuracy. Startups and financial institutions are already experimenting with limited versions of such technologies.
With its ability to process enormous amounts of historical data, AGI could create highly accurate financial models to assess risk and inform investment decisions. It could also develop and execute complex trading algorithms that incorporate market data, real-time news, and social media sentiment. However, human oversight would still be essential to ensure ethical considerations and to make the final decisions.
Example: A hedge fund employs an AGI system to monitor financial markets. The AGI detects a subtle shift in social media sentiment regarding a particular industry and flags a potential downturn. It cross-references this with historical data and news articles, confirming the likelihood of a market correction. Armed with this analysis, the fund manager can make informed adjustments to the portfolio, mitigating potential risks and capitalizing on emerging opportunities.
Research and Development
AGI could dramatically accelerate research and development by analyzing vast datasets, scientific literature, and generating innovative hypotheses at an unprecedented scale. Imagine having a scientific partner that not only examines data but also uncovers groundbreaking ideas by identifying subtle patterns and connections that human researchers might overlook. This capability could lead to the formulation of entirely new hypotheses and open up unexplored research avenues.
By simulating complex systems and managing vast amounts of data, AGI could design and conduct sophisticated experiments far more efficiently than current methods allow. This would enable scientists to test hypotheses rapidly and explore research frontiers that were previously unimaginable. AGI could tirelessly assist researchers in sifting through data, running complex simulations, and suggesting new directions for exploration, significantly accelerating the pace of scientific breakthroughs.
Example: A team of astrophysicists is studying the formation of galaxies in the early universe. The AGI system analyzes extensive datasets from telescopes and simulations, uncovering a previously unnoticed correlation between the distribution of dark matter and the formation of star clusters. Based on this discovery, the AGI proposes a new hypothesis about galaxy formation and suggests a series of innovative simulations to test its validity. This insight opens the door to a deeper understanding of the universe’s origins, pushing the boundaries of current scientific knowledge.
What Are the Types of AGI?
AGI promises to be a transformative technology, poised to revolutionize industries like healthcare and manufacturing. As large tech companies and research labs pour resources into its development, various approaches are being explored to achieve true human-level intelligence in machines. Here are some of the primary areas of exploration:
Symbolic AI: This approach focuses on creating systems that manipulate symbols and logic to represent knowledge and reasoning. It aims to build systems capable of understanding and solving problems by following rules, much like how humans use logic to navigate complex scenarios.
Connectionist AI (Artificial Neural Networks): Inspired by the structure and function of the human brain, this approach involves constructing artificial neural networks with interconnected nodes that learn and process information from vast amounts of data. This method mirrors how the human brain processes information and adapts over time.
Artificial Consciousness: This theoretical approach delves into giving machines subjective experiences and self-awareness. While still in the realm of speculation, artificial consciousness could be a critical component in achieving true AGI.
Whole Brain Emulation: This ambitious approach seeks to create a detailed computer simulation of a biological brain. The theory behind this is that by replicating the human brain’s structure and function, consciousness and intelligence might naturally emerge within the simulation.
Embodied AI and Embodied Cognition: This approach emphasizes the importance of an agent’s physical body and its interactions with the environment in shaping intelligence. The idea is that true intelligence cannot be fully realized without an agent experiencing and learning from the world through a physical body.
The field of AGI research is continually evolving, with these approaches representing just a fraction of the work being done. It’s likely that a combination of these techniques, or perhaps entirely new methodologies, will eventually lead to the realization of AGI.
Operationalizing AI: The Future of Business
While AGI remains a concept rooted in science fiction for now, organizations can begin preparing for the future by developing a robust AI strategy. By leveraging collaborative AI and data platforms, businesses can train, validate, tune, and deploy AI models, helping to scale and accelerate the impact of AI across the enterprise. Building a strong foundation today will position businesses to take full advantage of AI advancements as they continue to evolve.
It's never been easier to add Generative AI to your products. Explore how AI can benefit your business and how can our AI experts accelerate your development. Request a free consultation and discover our pool of talents that make your journey to AI easier.
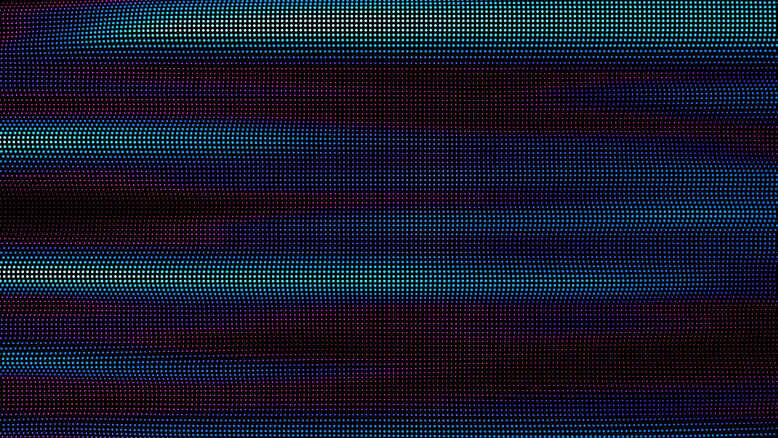