AI-powered agents are rapidly advancing beyond simple automation, offering unprecedented capabilities that can transform various sectors, from customer service to autonomous driving. Each type of AI agent, with its distinct approach to decision-making and interaction, represents a stepping stone toward more intelligent and autonomous systems. As these agents continue to evolve, understanding their functionalities, strengths, and limitations is crucial for businesses aiming to harness their full potential.
At the AI Forward 2023 conference, Bill Gates made a striking statement, emphasizing that the future of technology hinges on creating the most advanced AI agents. According to Gates, these AI agents could redefine our interactions with technology, to the point where traditional search engines or platforms like Amazon might no longer be necessary.
AI agents—intelligent virtual assistants powered by cutting-edge artificial intelligence—are designed to automate tasks, generate valuable insights, and enhance operational efficiency. These agents go beyond the basic functionalities of conventional voice assistants, taking on roles that range from digital coworkers to strategic partners in achieving business objectives. However, not all AI agents operate the same way!
Some are straightforward, others are highly sophisticated; some are proactive, while others are more utility-driven. There are those focused on learning and those with fixed functionalities.
In this article, we’ll dive into the various types of AI agents and explore their potential applications across different industries.
Key Takeaways:
• AI Agents are diverse, ranging from simple reflex-based systems to complex, learning-driven models, each suited to specific tasks and environments.
•can leverage different AI agent types to automate tasks, optimize processes, and enhance decision-making, leading to increased efficiency and innovation.
• Understanding the nuances of each AI agent type is essential for effectively integrating them into business operations, ensuring the right match between task complexity and agent capability.
• lies in the continued development and refinement of these agents, pushing the boundaries of what machines can achieve autonomously, while also emphasizing the need for ethical and responsible use.
Types of AI Agents
In the realm of Artificial Intelligence, agents are classified into various categories based on how their actions influence their intelligence and effectiveness. These categories include:
• Simple Reflex Agents
• Model-based Agents
• Goal-based Agents
• Utility-based Agents
• Learning Agents
• Hierarchical Agents
Understanding the unique characteristics of each agent type allows for optimizing their performance and enhancing decision-making processes. Let’s delve into the details of these AI agent types.
Simple Reflex Agent
A simple reflex agent operates by adhering to predefined rules, making decisions solely based on the present situation without considering past experiences or future outcomes.
This type of agent is particularly effective in environments where rules are consistent and actions are straightforward, as it relies entirely on reactive behavior to adapt to immediate changes in its surroundings.
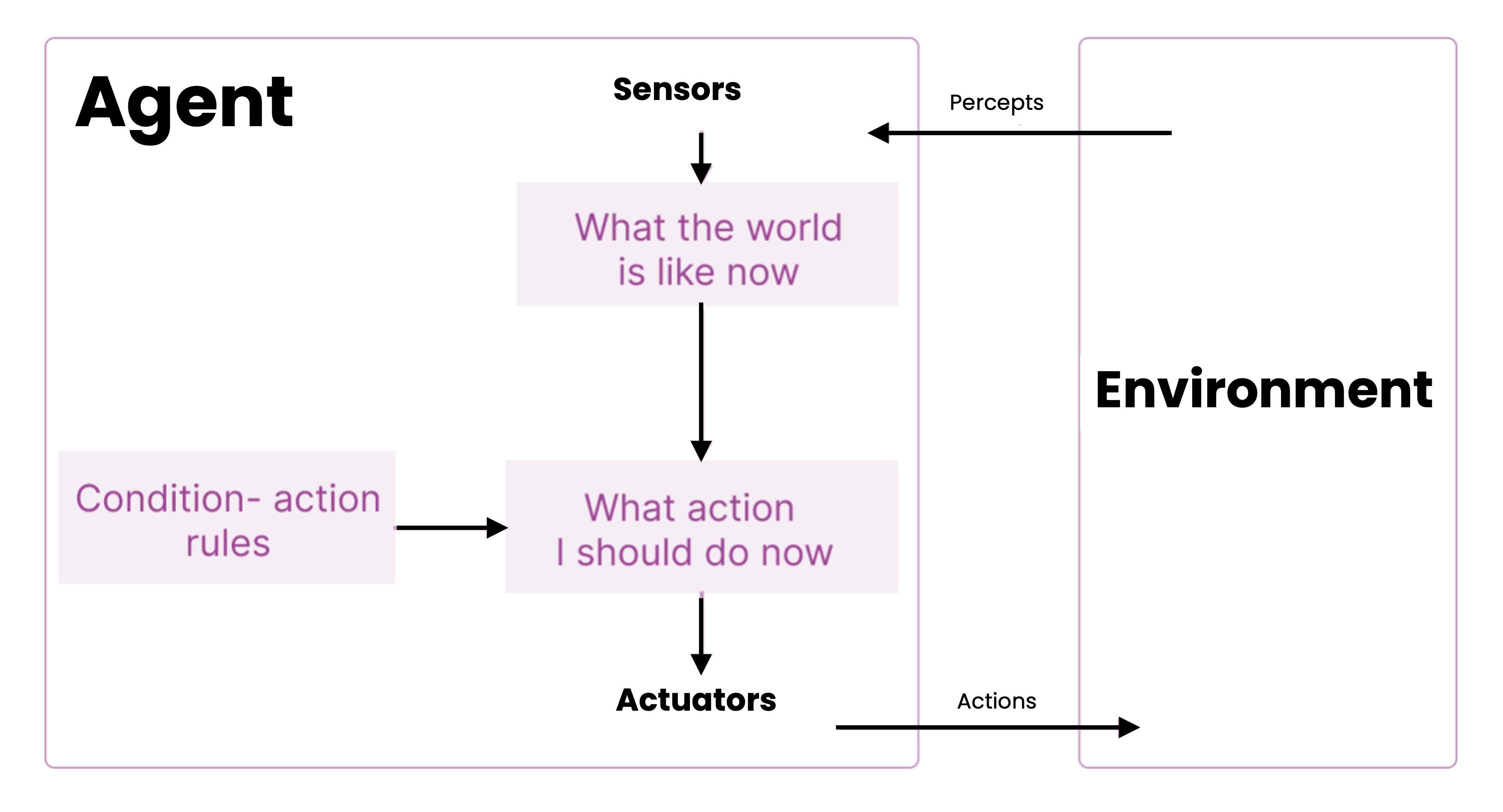
How It Works ?
The simple reflex agent functions by following a condition-action rule. This rule outlines specific actions to be taken when certain conditions are met.
Example
Consider a rule-based system designed for automated customer support. If a customer’s inquiry includes keywords related to password resetting, the system automatically provides a predefined response with instructions on how to reset the password.
Advantages of Simple Reflex Agents
• Easy to design and implement, requiring minimal computational resources.
• Capable of delivering real-time responses to changes in the environment.
• Highly reliable when input sensors are accurate and rules are well-constructed.
• No need for extensive training or sophisticated hardware.
Limitations of Simple Reflex Agents
• Prone to errors if input sensors malfunction or if the rules are poorly designed.
• Lack of memory or state, limiting their application scope.
• Incapable of handling situations where the environment is partially observable or undergoes unexpected changes.
• Restricted to a specific set of actions, making them inflexible and unable to adapt to new circumstances.
Model-based Agent
A model-based reflex agent acts based on its current perception and an internal representation of the unobservable aspects of the world. This agent continuously updates its internal state by considering two key factors:
• The independent evolution of the environment.
• The impact of the agent’s actions on the environment.
An advanced variant, known as a cautionary model-based reflex agent, takes this a step further by evaluating potential consequences before taking action.

How It Works?
While a model-based reflex agent follows a condition-action rule like a simple reflex agent, it goes beyond by utilizing an internal state to evaluate the current conditions before making decisions and taking actions.
The operation of a model-based reflex agent unfolds in four distinct stages:
• Sense: The agent gathers data from the environment through its sensors.
• Model: It builds an internal model of the world based on the sensory data.
• Reason: Using this internal model, the agent determines the best course of action by applying predefined rules or heuristics.
• Act: The agent then executes the chosen action.
Example
A prime example of a cautionary model-based reflex agent is Amazon Bedrock.
Amazon Bedrock leverages foundational models to simulate processes, derive insights, and make informed decisions, enhancing planning and optimization. Through its various models, Bedrock can predict outcomes, refine its operations with real-time data, and adjust strategies dynamically by simulating different scenarios and modifying model parameters.
Advantages of Model-based Reflex Agents
• Efficient Decision-Making: They make quick and accurate decisions by drawing on their comprehensive understanding of the environment.
• Enhanced Accuracy: By constructing an internal model, these agents are better positioned to make precise decisions.
• Adaptability: Their ability to update internal models allows them to adapt to changes in the environment.
• Strategic Thinking: They make more informed decisions by leveraging their internal state and rules.
Disadvantages of Model-based Reflex Agents
• High Computational Costs: Developing and maintaining these models can be resource-intensive.
• Incomplete Environmental Representation: The models may not fully capture the complexities of real-world environments.
• Unpredictability: They may struggle with unanticipated situations that the models do not account for.
• Frequent Updates Needed: To remain effective, models must be regularly updated.
• Interpretation Challenges: The complexity of these models can make them difficult to interpret and understand.
Goal-based Agent
Goal-based agents are AI-driven systems designed to achieve specific objectives by leveraging information from their environment. They utilize search algorithms to identify the most efficient paths toward their goals within a given context.
These agents are sometimes referred to as rule-based agents because they operate according to predefined rules that guide their actions based on particular conditions.
Goal-based agents are relatively straightforward to design and are capable of managing complex tasks. They find applications across various fields, including robotics, computer vision, and natural language processing.
Unlike simpler models, goal-based agents can assess the optimal strategies for decision-making and actions by focusing on their defined outcomes or objectives.
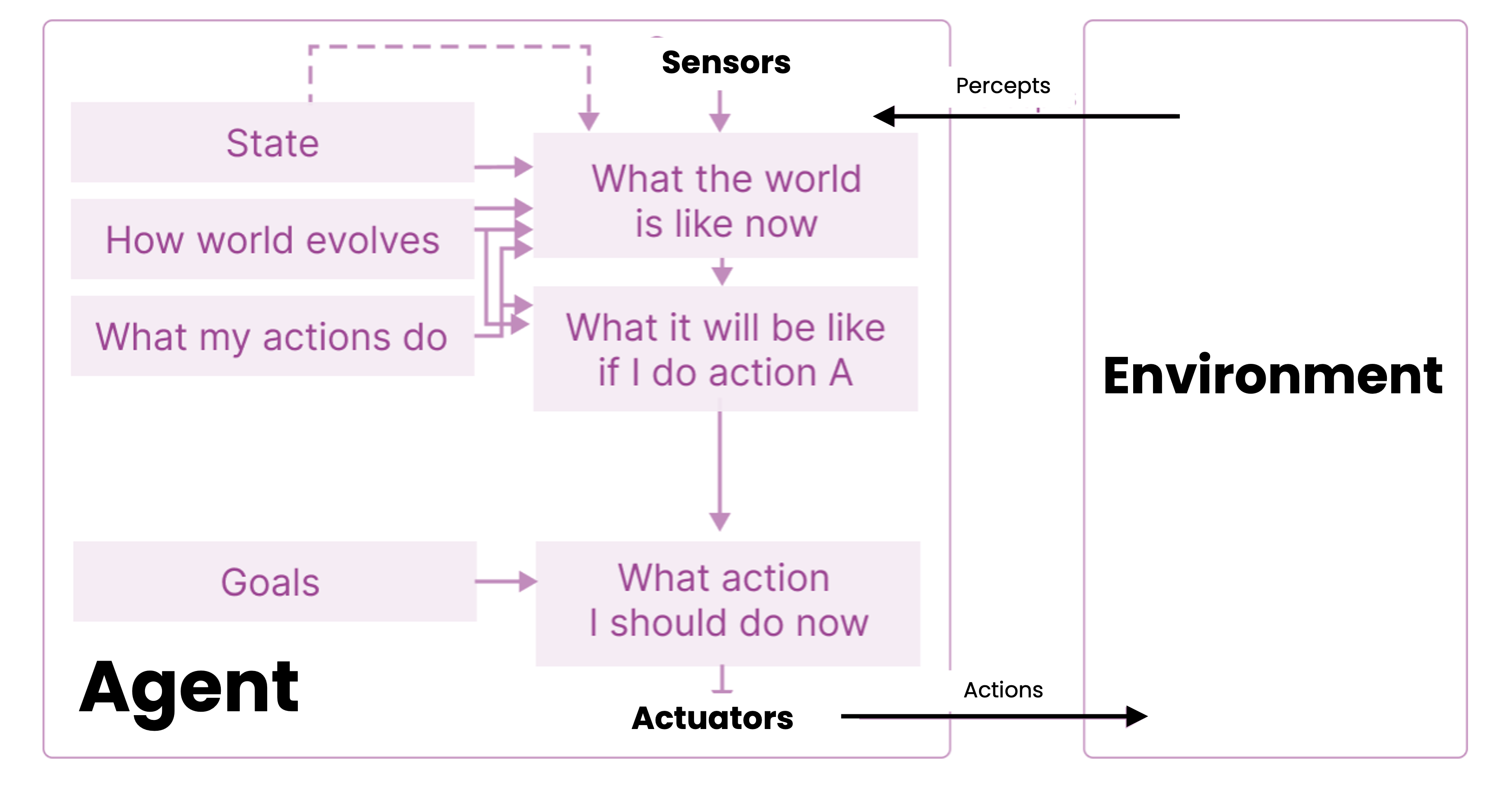
How It Works?
When presented with a plan, a goal-based agent works to select the best strategy to achieve its goals. It does this by employing search algorithms and heuristics to determine the most efficient route to its objective.
The operation of a goal-based agent can be broken down into five key steps:
• Perception: The agent gathers data from its surroundings through sensors or other input devices.
• Reasoning: The agent analyzes this information to determine the most effective course of action to meet its goal.
• Action: The agent executes the necessary actions, such as moving or interacting with objects in its environment, to progress toward its goal.
• Evaluation: Following the action, the agent assesses its progress and makes any necessary adjustments to stay on track.
• Goal Completion: Once the agent achieves its goal, it either halts its activity or begins working toward a new objective.
Example
OpenAI Chat Assistant can be considered a goal-based agent, though it also functions as a learning agent. As a goal-based agent, its primary objective is to deliver high-quality responses to user inquiries. It selects actions that are most likely to help users find the information they seek, thus fulfilling the goal of providing accurate and useful answers.
Advantages of Goal-based Agents
• Ease of Implementation: Simple to design and understand.
• Goal Efficiency: Effective at achieving specific objectives.
• Performance Evaluation: Easy to measure success based on goal completion.
• Versatility: Can be combined with other AI techniques to create more sophisticated agents.
• Suitability: Well-matched for structured, well-defined environments.
• Broad Application: Applicable in various domains, such as robotics, game AI, and autonomous vehicles.
Disadvantages of Goal-based Agents
• Goal Limitation: Restricted to pursuing a single specific goal.
• Environmental Rigidity: Struggles to adapt to dynamic or changing environments.
• Complex Task Challenges: Less effective for tasks with numerous variables.
• Domain Knowledge Requirement: Requires substantial domain expertise to define effective goals.
Utility-based Agents
Utility-based agents are AI systems designed to make decisions by maximizing a utility function or value, allowing them to select the action with the highest expected utility—essentially, the best possible outcome.
These agents are particularly adept at navigating complex and uncertain environments, as they can flexibly and adaptively choose among multiple options. Utility-based agents are commonly employed in scenarios where they need to evaluate and prioritize several alternatives, such as in resource allocation, scheduling, and strategic game-playing.
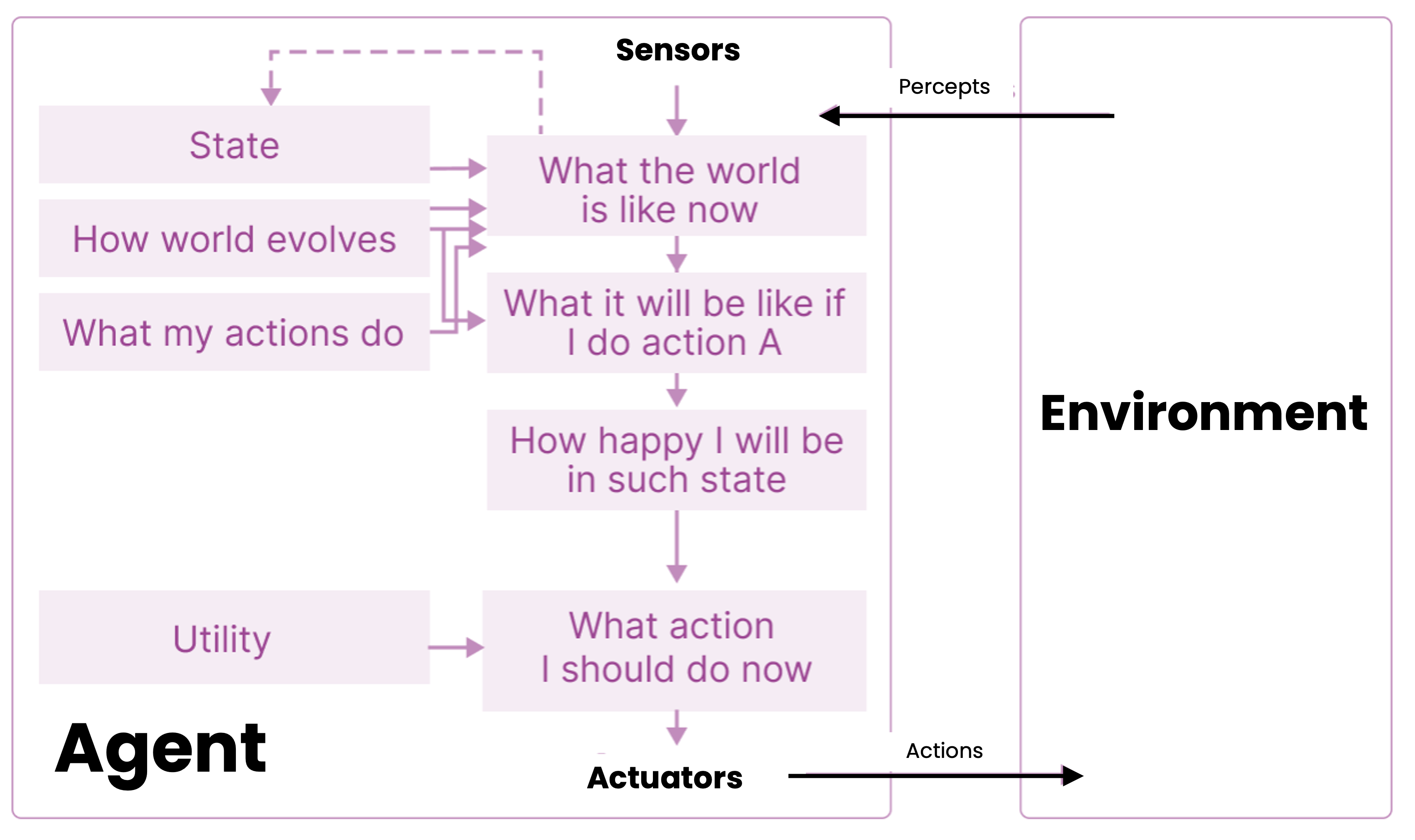
How It Works?
The primary goal of a utility-based agent is to take actions that lead to a state of high utility. To do this, the agent first models its environment, which can range from simple to highly complex.
Next, it assesses the expected utility of each potential outcome, using a probability distribution and a utility function to quantify the desirability of each outcome.
Finally, the agent selects the action that promises the highest expected utility and repeats this process continuously at each decision point.
Example
Anthropic Claude powering an AI Agent serves as an excellent example of a utility-based agent. Its primary objective is to assist cardmembers in maximizing their rewards and benefits from using credit cards.
To achieve this, Claude utilizes a utility function that assigns numerical values to different outcomes, such as making purchases, paying bills, or redeeming rewards. The agent then compares the potential results of different actions in each scenario, making trade-offs based on the calculated utility values.
Moreover, Claude incorporates heuristics and advanced AI techniques to streamline and enhance the decision-making process.
Advantages of Utility-based Agents
• Versatile Decision-Making: Capable of handling a broad spectrum of decision-making challenges.
• Adaptive Learning: Able to learn from past experiences and refine decision strategies accordingly.
• Objective Framework: Provides a consistent and objective method for making decisions.
Disadvantages of Utility-based Agents
• Environmental Dependence: Requires a highly accurate model of the environment, and any inaccuracies can lead to poor decision-making.
• High Computational Cost: Demands extensive calculations, which can be computationally intensive.
• Ethical Blind Spots: Does not inherently consider moral or ethical implications in its decision-making process.
• Complexity: Can be difficult for humans to understand and validate the reasoning behind its decisions.
Learning Agents
A learning agent is an AI-driven system that can evolve and enhance its performance by learning from past experiences. Initially, it operates with a basic level of knowledge, but over time, it adapts and improves autonomously through machine learning techniques.
A learning agent consists of four core components:
• Learning Element: Responsible for acquiring knowledge and refining its actions based on the experiences gained from its environment.
• Critic: Provides feedback on the agent’s performance, comparing it to a predefined standard to guide improvements.
• Performance Element: Executes external actions by utilizing the knowledge provided by the learning element and the feedback from the critic.
• Problem Generator: Suggests new actions to the learning element to create informative experiences that drive further learning and performance enhancement.

How It Works?
Learning agents operate in a continuous cycle of observation, learning, action, and feedback. They engage with their environment, gather data from their actions, and use feedback to adjust their behavior for better outcomes in the future.
Here’s how the cycle typically unfolds:
• Observation: The agent monitors its environment using sensors or other input mechanisms to collect relevant data.
• Learning: It processes this data using algorithms and statistical models, learning from the feedback it receives on its performance and decisions.
• Action: Armed with new insights, the agent takes actions within its environment, making informed decisions on how to proceed.
• Feedback: The agent receives feedback—whether in the form of rewards, penalties, or environmental signals—about the effectiveness of its actions.
• Adaptation: The agent updates its behavior and decision-making processes based on the feedback, refining its approach to future interactions.
Hierarchical Agents
Hierarchical agents are structured in a layered system, where high-level agents oversee and manage lower-level agents. The number of levels in the hierarchy can vary depending on the complexity of the system.
These agents are particularly effective in applications such as robotics, manufacturing, and transportation, where they excel at coordinating and prioritizing multiple tasks and sub-tasks simultaneously.
How It Works
Hierarchical agents function much like a corporate organization, where tasks are organized into a structured hierarchy. Higher-level agents are responsible for overseeing the entire process, breaking down overarching goals into smaller, manageable tasks.
These smaller tasks are then delegated to lower-level agents, who execute them and report their progress back up the chain.
In more complex systems, there might be intermediate-level agents that coordinate the activities between the lower-level and higher-level agents, ensuring smooth communication and task execution across all levels.
Example
An excellent example of a hierarchical AI agent is Google’s UniPi. UniPi leverages text and video as a universal interface, enabling it to learn and perform a variety of tasks across different environments.
UniPi operates with a high-level policy that is responsible for generating instructions and demonstrations, while the low-level policy handles the execution of these tasks. The high-level policy can adapt to various environments and tasks, while the low-level policy learns through techniques like imitation and reinforcement learning.
This hierarchical framework allows UniPi to effectively combine strategic, high-level reasoning with detailed, low-level execution.
Advantages of Hierarchical Agents
• Resource Efficiency: By assigning tasks to the most appropriate agents, hierarchical systems minimize duplication of effort, making them resource-efficient.
• Enhanced Communication: Clear lines of authority and direction within the hierarchy improve overall communication.
• Improved Decision-Making: Hierarchical Reinforcement Learning (HRL) simplifies complex decisions by breaking them down into more manageable high-level actions, facilitating exploration and learning.
• Reduced Computational Complexity: Hierarchical decomposition makes problems more manageable by representing them in a concise, reusable format, which helps in reducing computational demands.
Disadvantages of Hierarchical Agents
• Complexity in Problem-Solving: The use of hierarchies can introduce complexity when tackling problems, making them harder to solve.
• Limited Adaptability: Fixed hierarchies can hinder the agent’s ability to adapt to changing or unpredictable environments, reducing flexibility.
• Bottlenecks: The top-down control structure may lead to bottlenecks and delays, especially when lower-level tasks are ready but awaiting higher-level approval.
• Domain-Specific Limitations: Hierarchies often lack reusability across different domains, necessitating the creation of new hierarchies for each specific domain, which can be both time-consuming and require specialized expertise.
• Challenging Training Process: Training hierarchical agents is complex, requiring labeled data and careful algorithm design. Standard machine learning techniques are often difficult to apply due to the intricate nature of hierarchical systems.
Real-life Examples of AI Agents
• Zendesk AI: Zendesk has introduced an AI-driven solution for intelligent customer experience (CX) management, featuring advanced bots, agent assistance, and intelligent triage. These capabilities enable more effective and efficient customer service by facilitating smarter conversations that quickly resolve issues. For example, Zendesk AI can suggest responses, detect customer intent, and ensure that agents are well-prepared and refreshed for each interaction.
• AI Sandbox: Meta’s AI Sandbox is an innovative platform designed for advertisers to experiment with new generative AI tools. It offers features such as alternative text variations, background generation, and image cropping for Facebook and Instagram ads. These tools empower brands to effortlessly create diverse ad copy, generate unique visual assets, and design content that fits various formats, from social media posts to short videos.
• Gemini: Google’s Gemini AI represents the next generation of large language models (LLM), incorporating reinforcement learning inspired by AlphaGo. Gemini enhances its problem-solving abilities by rewarding positive behaviors and correcting mistakes, allowing it to learn independently from its actions and the feedback it receives, without needing direct human supervision.
• Speak AI: Speak AI provides automated transcription services that convert audio and video content into text. It offers an embeddable recorder for capturing recordings and integrates with popular platforms to streamline the capture process. Users can ask questions and receive meaningful responses through pre-designed speech prompts. Additionally, Speak AI supports various integrations, including Zapier, Google Chrome Extension, Zoom, and Vimeo, to automate workflows and enhance productivity.
• Camel AGI: Camel AGI is an agent framework designed to guide chat agents towards completing tasks by role-playing, ensuring consistency with human intentions. It enhances dialogue systems, develops interactive gaming characters, simulates expert discussions, and addresses complex problems across various fields. Camel AGI also plays a crucial role in exploring conversational language models, making it a versatile tool in AI research and development.
Conclusion
As AI technology advances, AI agents are poised to become increasingly autonomous, capable of making independent decisions with minimal human oversight. These agents have the potential to revolutionize various industries by automating customer service, forecasting demand, optimizing production processes, and much more.
The possibilities for AI agents across different sectors are vast and hold immense promise for driving innovation and efficiency.
However, it is essential to approach the deployment of AI agents with a strong ethical framework, ensuring that their use benefits both your enterprise and society as a whole.
Therefore, it’s important to make informed decisions and strategically embrace the power of AI agents to unlock their full potential for your business.
It's never been easier to add Generative AI to your products. Explore how AI can benefit your business and how can our AI experts accelerate your development. Request a free consultation and discover our pool of talents that make your journey to AI easier.
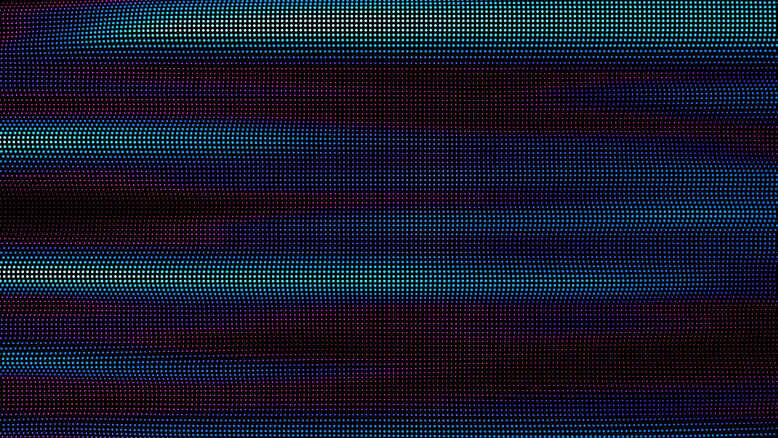